Using callbacks in objectives¶
This notebook explains how to use a callback in an objective function. Potential use cases for this are:
Plotting some outputs at each iteration of the optimization
Saving internal variables to plot once the optimization is complete
Some objectives have “internal callbacks” which are not intended to be user facing. These are standard callbacks that can be used to plot the results of an optimization by using DataFit.plot_fit_results()
. For user-facing callbacks, users should create their own callback objects and call them directly for plotting, as demonstrated in this notebook
Creating a custom callback¶
To implement a custom callback, we create a class that inherits from iwp.callbacks.Callback
and calls some specific functions. See the documentation for iwp.callbacks.Callback
for more information on the available functions and their expected inputs.
import ionworkspipeline as iwp
import matplotlib.pyplot as plt
import pybamm
import numpy as np
import pandas as pd
class MyCallback(iwp.callbacks.Callback):
def __init__(self):
super().__init__()
# Implement our own iteration counter
self.iter = 0
def on_objective_build(self, logs):
self.data_ = logs["data"]
def on_run_iteration(self, logs):
# Print some information at each iteration
inputs = logs["inputs"]
V_model = logs["outputs"]["Voltage [V]"]
V_data = self.data_["Voltage [V]"]
# calculate RMSE, note this is not necessarily the cost function used in the optimization
rmse = np.sqrt(np.nanmean((V_model - V_data) ** 2))
print(f"Iteration: {self.iter}, Inputs: {inputs}, RMSE: {rmse}")
self.iter += 1
def on_datafit_finish(self, logs):
self.fit_results_ = logs
def plot_fit_results(self):
"""
Plot the fit results.
"""
data = self.data_
fit = self.fit_results_["outputs"]
fit_results = {
"data": (data["Time [s]"], data["Voltage [V]"]),
"fit": (fit["Time [s]"], fit["Voltage [V]"]),
}
markers = {"data": "o", "fit": "--"}
colors = {"data": "k", "fit": "tab:red"}
fig, ax = plt.subplots()
for name, (t, V) in fit_results.items():
ax.plot(
t,
V,
markers[name],
label=name,
color=colors[name],
mfc="none",
linewidth=2,
)
ax.grid(alpha=0.5)
ax.set_xlabel("Time [s]")
ax.set_ylabel("Voltage [V]")
ax.legend()
return fig, ax
To use this callback, we generate synthetic data for a current-driven experiment and fit a SPM using the CurrentDriven
objective.
model = pybamm.lithium_ion.SPM()
parameter_values = pybamm.ParameterValues("Chen2020")
sim = pybamm.Simulation(model, parameter_values=parameter_values)
sim.solve(np.linspace(0, 3600, 1000))
data = pd.DataFrame(
{x: sim.solution[x].entries for x in ["Time [s]", "Current [A]", "Voltage [V]"]}
)
# In this example we just fit the diffusivity in the positive electrode
parameters = {
"Positive particle diffusivity [m2.s-1]": iwp.Parameter("D_s", initial_value=1e-15),
}
# Create the callback
callback = MyCallback()
objective = iwp.objectives.CurrentDriven(
data, options={"model": model}, callbacks=callback
)
current_driven = iwp.DataFit(objective, parameters=parameters)
# make sure we're not accidentally initializing with the correct values by passing
# them in
params_for_pipeline = {k: v for k, v in parameter_values.items() if k not in parameters}
params_fit = current_driven.run(params_for_pipeline)
Iteration: 0, Inputs: {'D_s': 1.0}, RMSE: 0.15909544741400455
Iteration: 1, Inputs: {'D_s': 1.0}, RMSE: 0.15909544741400455
Iteration: 2, Inputs: {'D_s': 2.0}, RMSE: 0.06447645367955734
[IDAS ERROR] IDACalcIC
The linesearch algorithm failed: step too small or too many backtracks.
[IDAS ERROR] IDASolve
At t = 0 and h = 8.88112e-60, the corrector convergence failed repeatedly or with |h| = hmin.
Iteration: 3, Inputs: {'D_s': 0.0}, RMSE: 9999999996.444778
Iteration: 4, Inputs: {'D_s': 1.500000000015711}, RMSE: 0.1018141923039706
Iteration: 5, Inputs: {'D_s': 2.25}, RMSE: 0.05119146930398495
Iteration: 6, Inputs: {'D_s': 2.35}, RMSE: 0.04656481722357566
Iteration: 7, Inputs: {'D_s': 2.45}, RMSE: 0.042256791629341615
Iteration: 8, Inputs: {'D_s': 2.5500000000000003}, RMSE: 0.038236231397813014
Iteration: 9, Inputs: {'D_s': 2.6500000000000004}, RMSE: 0.034472752119072594
Iteration: 10, Inputs: {'D_s': 2.79142135623731}, RMSE: 0.029545925327789383
Iteration: 11, Inputs: {'D_s': 2.89142135623731}, RMSE: 0.026301971986168914
Iteration: 12, Inputs: {'D_s': 2.99142135623731}, RMSE: 0.023247195931469865
Iteration: 13, Inputs: {'D_s': 3.13284271247462}, RMSE: 0.019211881023240635
Iteration: 14, Inputs: {'D_s': 3.33284271247462}, RMSE: 0.014012123861796226
Iteration: 15, Inputs: {'D_s': 3.43284271247462}, RMSE: 0.011609786833907025
Iteration: 16, Inputs: {'D_s': 3.5328427124746202}, RMSE: 0.009325897899196063
Iteration: 17, Inputs: {'D_s': 3.67426406871193}, RMSE: 0.006282677069898297
Iteration: 18, Inputs: {'D_s': 3.8273301846063092}, RMSE: 0.003212744846976506
Iteration: 19, Inputs: {'D_s': 3.9273301846063093}, RMSE: 0.0013216783239264063
Iteration: 20, Inputs: {'D_s': 4.027330184606309}, RMSE: 0.0004855748677146316
Iteration: 21, Inputs: {'D_s': 4.168751540843618}, RMSE: 0.0029061820438003537
Iteration: 22, Inputs: {'D_s': 4.092094987762795}, RMSE: 0.0016113565966859556
Iteration: 23, Inputs: {'D_s': 4.055594668968367}, RMSE: 0.0009794598019568425
Iteration: 24, Inputs: {'D_s': 4.002330184606309}, RMSE: 4.213938330197731e-05
Iteration: 25, Inputs: {'D_s': 3.9773301846063087}, RMSE: 0.00040803108661856746
Iteration: 26, Inputs: {'D_s': 4.012330184606308}, RMSE: 0.00021972444774458484
Iteration: 27, Inputs: {'D_s': 3.992330184606309}, RMSE: 0.0001380644177823006
Iteration: 28, Inputs: {'D_s': 4.007277439244583}, RMSE: 0.00012985611023525438
Iteration: 29, Inputs: {'D_s': 3.9998301846063087}, RMSE: 9.122720180680779e-06
Iteration: 30, Inputs: {'D_s': 3.9973301846063087}, RMSE: 4.8898026626834985e-05
Iteration: 31, Inputs: {'D_s': 3.998830184606309}, RMSE: 2.292519090088247e-05
Iteration: 32, Inputs: {'D_s': 4.000830184606309}, RMSE: 1.676251684096855e-05
Iteration: 33, Inputs: {'D_s': 4.0003299567887325}, RMSE: 1.01050900963485e-05
Iteration: 34, Inputs: {'D_s': 3.999580184606309}, RMSE: 1.1565004187323774e-05
Iteration: 35, Inputs: {'D_s': 4.000017275322229}, RMSE: 8.460568714634666e-06
Iteration: 36, Inputs: {'D_s': 4.0001172753222285}, RMSE: 8.633825402874301e-06
Iteration: 37, Inputs: {'D_s': 4.000007275322229}, RMSE: 8.463860978345447e-06
Iteration: 38, Inputs: {'D_s': 4.000027275322228}, RMSE: 8.46105703367714e-06
Iteration: 39, Inputs: {'D_s': 4.000020983672041}, RMSE: 8.460308691780029e-06
Iteration: 40, Inputs: {'D_s': 4.000021983672041}, RMSE: 8.460327591497893e-06
Iteration: 41, Inputs: {'D_s': 4.000019983672041}, RMSE: 8.460327605175042e-06
Iteration: 42, Inputs: {'D_s': 4.000020983672041}, RMSE: 8.460308691780029e-06
Now we use the callback object we created to plot the results at the end of the optimization.
callback.plot_fit_results()
(<Figure size 640x480 with 1 Axes>,
<Axes: xlabel='Time [s]', ylabel='Voltage [V]'>)
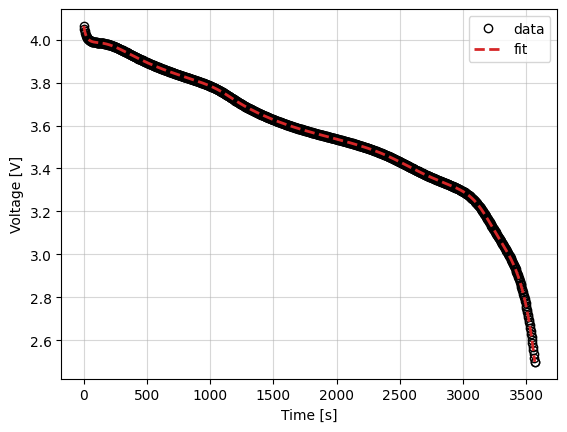
Cost logger¶
The DataFit
class has an internal “cost-logger” attribute that can be used to log and visualize the cost function during optimization. This is useful for monitoring the progress of the optimization. The cost logger is a dictionary that stores the cost function value at each iteration. The cost logger can be accessed using the cost_logger
attribute of the DataFit
object.
By default, the cost logger just tracks the cost function value. DataFit.plot_trace
can be used the plot the progress at the end of the optimization.
objective = iwp.objectives.CurrentDriven(data, options={"model": model})
current_driven = iwp.DataFit(objective, parameters=parameters)
params_fit = current_driven.run(params_for_pipeline)
current_driven.plot_trace()
[IDAS ERROR] IDACalcIC
The linesearch algorithm failed: step too small or too many backtracks.
[IDAS ERROR] IDASolve
At t = 0 and h = 8.88112e-60, the corrector convergence failed repeatedly or with |h| = hmin.
(<Figure size 800x400 with 2 Axes>,
array([<Axes: xlabel='Iteration', ylabel='Cost'>,
<Axes: xlabel='Iteration', ylabel='D_s'>], dtype=object))
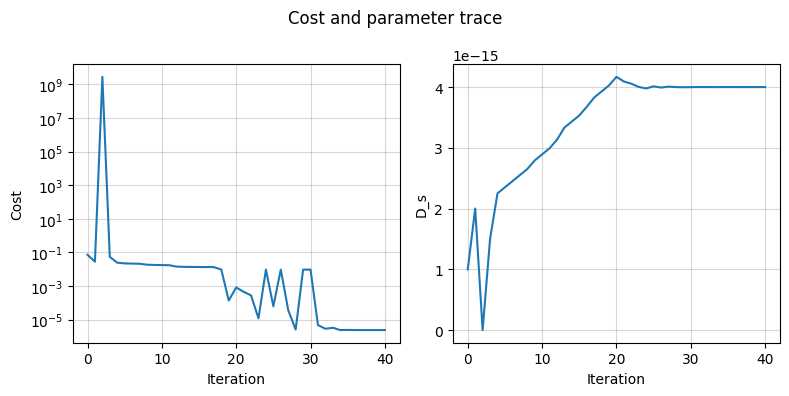
The cost logger can be changed by passing the cost_logger
argument to the DataFit
object. For example, the following example shows how to pass a cost logger that plots the cost function and parameter values every 10 iterations.
current_driven = iwp.DataFit(
objective,
parameters=parameters,
cost_logger=iwp.data_fits.CostLogger(plot_every=10),
)